
Researchers and growers explore the use of drones in nursery production.
The popularity of drones, aka unmanned aerial vehicles or systems (UAVs/UASs), has dramatically increased over the past decades.
Like global positioning (GPS) technology, walkie-talkies and Jeeps, drones initially were developed for military use. Although the U.S. Air Force’s Predator and Reaper drones continue to appear on the evening news, and as a plot device in action movies, it is not likely that one will whirl into your life from the hobbyist market.
The rapid growth of hobby drone sales prompted concerns about safety and aerial congestion. This concern resulted in the Federal Aviation Administration (FAA) setting up a registration system for all drones, both for commercial and hobby use (see www.faa.gov for details). The FAA’s vision was for the full integration of UASs into the National Airspace System to operate harmoniously with manned aircraft, using many of the same air traffic management systems and procedures. While little winged copters have become common gifts and can be regularly seen patrolling parks or parties, the non-military application of drones is not just for Hollywood or holidays. In fact, civil and commercial industries are being recognized as the largest emerging drone market.
The Goldman Sachs investment bank is projecting $100 billion in UAV market growth over the next two years. Agriculture is one of the larger commercial sectors for drone applications. Within agriculture, drones have been used to locate livestock, deploy agri-chemicals (i.e., spraying), and achieve a variety of other purposes.
Good tool for agriculture
Currently, the two primary applications for drones in specialty crops are for crop health monitoring and survey applications.
Crop health monitoring is one of the most commonly marketed applications of drones in agriculture. Images collected during flight can be used to monitor crop health across large areas with few people. Drones offer an improvement from manned aerial vehicles (i.e., small airplanes) or LANDSAT imagery because drones can provide increased flight frequency and better image quality from low-level flights.
Many agricultural drone software platforms alluringly display colorimetric approaches to crop health monitoring in green to red spectrums on their websites. For colorimetric approaches, aerial images can be analyzed in visible wavelengths, and the images can be contrasted in multiple wavelengths to detect non-visible signals. For example, the normalized difference vegetation index (NDVI) is a ratio at the core of many proprietary software platforms offered in the agricultural market.
The fundamentals of NDVI relate to light wavelength and light reflectance. The presence of chlorophyll in the plant tissues causes absorption of red light wavelengths. Non-green plant structures and biomass reflect relatively more near-infrared (NIR) wavelengths.
In general, healthy plants in dense canopies reflect high levels of NIR and have low red light reflectance. On the other hand, when there are few plants or unhealthy plants more red light is reflected. The NDVI is a ratio of this phenomenon NDVI = NIR – Red / NIR + Red. For healthy plants and dense vegetation, the ratio will tend towards +1.
On the other hand, unhealthy crops will reflect more red light and have a lower NDVI value. Brayden Sechser trialed a colorimetric approach at Kraemer’s Nursery Inc. (KNI). For Sechser, who flew a fixed-wing drone, the hope was that UAV would support KNI’s crop health team by indicating specific locations that needed more attention.
When small teams are expected to monitor large acreages, spotting problems can be like finding a needle in a haystack. For example, a malfunctioning emitter or head could go unreported for a few days when there are hundreds or thousands of emitters in an irrigation system — especially if irrigation is timed to run at off-hours, allowing for crew access during work hours. As any grower knows, missed irrigation on full-grown plants in the heat of the summer can be a costly and deadly error.
KNI’s team used the drone flights with early identification methods. “The cameras can sometimes detect problems before we can see them,” said Sechser. Oregon State University (OSU) researchers are also applying colorimetric analysis methods in grape vineyards in an attempt to detect plant pathogen outbreaks. The OSU Aerial Information Systems Laboratory uses infrared technology to spot the presence of grapevine red blotch virus and potentially other viruses before they are noticeable to human eyes.
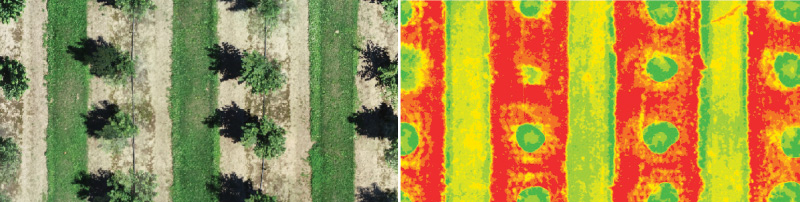
(Left) Aerial view of trees on a nursery as seen with the naked eye. (Right) Colorimetric renderings look at plant health from a different lens.
Photos courtesy of Oregon State University
Field surveys are another typical application for UAV. Over the past few years, OAN members have supported a few different drone research projects with nursery and Christmas tree applications, thanks to funding from the ODA Nursery Research Grant Program.
In 2010, OSU researchers partnered with researchers from the University of Arkansas and University of Florida to develop a cost-effective, low altitude aerial imagery system to automate inventory processes at shade tree nurseries, container nurseries and Christmas tree farms. The study operated under the premise that collecting real-time inventory data is expensive, time-consuming and can be imprecise.
Their project assumed that semi-automating the inventory process with UAV would reduce labor inputs, increase count accuracy, reduce workforce strain, and reduce overall costs. An additional benefit was that the drone imagery could simultaneously map roads, buildings, irrigation and other undocumented infrastructure.
Eight years ago, when drones were new to the market, the research project objectives included basic evaluations of sensors and environmental factors that would influence image quality. The researchers learned that several factors contributed to the complexity of imagery used for plant inventory analysis, including plant characteristics (plant color, species, plant size and shape, canopy cover, plant health), ground and surface characteristics (bare soil, gravel, ground cloth), and environmental factors (sunlight, shadows).
One of the major hurdles was that plant canopies sometimes hampered the ability to discern individual plants. Late in the season as plant canopies filled out both in-containers and in-field facilities, it was challenging for the software (or often even the individual eye) to determine where one plant’s branches stopped and another’s began.
To address this, in the later years of the project, the research team began investigating how spatial resolution and canopy separation on different ground surfaces affected image analysis algorithms. The team was eventually able to achieve 98 percent accuracy in a count of 22,000 plants with drone-collected images, including plants with overlapping canopies.
However, like almost everything else in the nursery business, there is no one-size-fits-all solution. Inventory algorithms and some plant scenarios remain challenging.
Site-specific variables, as well as the diverse range of plants grown by various nurseries, may require nursery-specific or even block-specific counting algorithms.
J. Frank Schmidt & Son Co. (JFS) has been a voluntary test site on many of these projects and is hopeful that drones could eventually help count the several million plants they have in the ground. Although progress has been made counting large broad areas, JFS is hopeful for a practical, cost-effective, row-by-row counting solution.
Issues with stitching — the merging of hundreds of smaller photos generated during flight into one large photo — can result in misaligned counts of trees in a row. For now, this is a technical problem that can result in a significant miscalculation of available product.
Similarly, Sechser at KNI also has high hopes for drone-based inventory analysis. In his estimation, the inventory software has not been developed sufficiently enough to be solely reliable for inventory counts. In the yards he manages, where product frequently moves with different spacing, there are concerns with the ability to distinguish individual units — especially in can-tight or closed canopy situations.
In discussions with software developers, Sechser has learned that large datasets are still required to refine the counting algorithms. While the technology is rapidly improving it is not yet grower-ready.
The future of flight
For all the perceived opportunities of incorporating drone technologies, there are still some barriers to adoption in nursery crop systems. In addition to the previously mentioned inventory issues, there are also crop-health challenges with colorimetric approaches (e.g., NDVI).
In a sense, NDVI is a measure of “greenness” and has an established application in field crops like corn, soy, and other agronomic varieties. The utility of “greenness” is lesser known for nursery crops. We work with such a wide variety of plant types within and between nurseries that specific crop- or variety-specific detail rarely exists.
For instance, the relevant NDVI range for variegated, glaucous, and highly pigmented young or mature leaves is unknown. The spectral signature for species with colored leaves can be different from uniformly green crops. Uncorrelated spectral signatures present a problem when green and non-green crops grow adjacent to each other. Like green crops, colored leaves will emit a reflectance signature that will change in response to crop health. However, at this time, the ‘danger’ thresholds are unknown for most nursery species.
A second barrier is a cost. Entering the drone market carries capital and operating expenses. It is our experience that a commercial research-level drone with a high-quality camera will cost $10,000–20,000 initially, with potentially $1,000–3,000 in annual software fees. These costs exclude any required maintenance and repairs when — not if — a collision happens.
The return on investment is challenging to predict given the many unknowns with the developing technology.
As time goes by, the efficacious use of drones has become closer to reality. Inventory algorithms continue to be refined and high-quality sensors are becoming ubiquitous. Like the investment banks who predict billions of dollars of growth over the next two years, OSU also recognizes the significant value of UAV to support specialty crop growers — especially when growers have fewer eyes available to monitor crop health and inventory.
Therefore, the OSU Nackley Lab has recently partnered with Dr. Kristine Buckland, assistant professor and vegetable and specialty seed crop specialist, to develop a drone-based research program at OSU’s North Willamette Research and Extension Center. The initial goals are twofold.
First, we wish to guide growers who are interested in entering the market, but who are overwhelmed by the initial costs and variety of similar products available in the drone market. Secondly, Dr. Nackley’s program will address the fundamental lack of knowledge of ornamental crops.
He has partnered with Dr. Carolyn Scagel, USDA ARS Horticultural Crops Research Unit, to calibrate nutrient stress with colorimetric approaches from multi-spectral images in nursery production systems. They plan to expand the program to include to water stress identification as well.
OSU researchers will continue to develop partnerships with growers and other universities to inform and assist technological incorporation of technology like UAVs in Oregon nursery production systems.
Lloyd Nackley is an assistant professor of nursery and greenhouse production systems at Oregon State University (OSU). He can be reached at [email protected]. Heather Stoven is an assistant professor (practice) of community horticulture and small farms at OSU. She can be reached at [email protected].